In today’s data-driven world, companies are constantly searching for ways to leverage the vast amounts of information they collect to gain a competitive edge. Big data analytics has emerged as a powerful tool for extracting valuable insights from this data, enabling businesses to make informed decisions, optimize operations, and improve customer experiences. However, handling and processing such massive datasets requires robust infrastructure, leading to a growing demand for reliable and efficient server solutions. As we step into 2024, it’s crucial to understand the best server solutions designed specifically for big data analytics. This comprehensive guide will explore the top server options available, highlighting their unique features, benefits, and suitability for various data analytics needs.
The Growing Importance of Big Data Analytics
In today’s rapidly evolving digital landscape, data is king. Businesses are generating massive amounts of data every day, and the ability to analyze and extract meaningful insights from this data has become paramount. Big data analytics, the process of examining large, complex datasets to uncover hidden patterns, trends, and correlations, has emerged as a critical driver of success for organizations across industries.
The rise of big data analytics is driven by several key factors. First, the proliferation of connected devices, such as smartphones, wearables, and sensors, is generating an unprecedented volume of data. Second, the increasing availability of cloud computing and storage solutions has made it easier and more affordable for businesses to store and process vast amounts of data. Finally, the advancements in machine learning and artificial intelligence (AI) algorithms have enabled organizations to extract insights from data more efficiently and effectively.
The benefits of big data analytics are numerous and far-reaching. Businesses can leverage this technology to:
- Improve customer experience and personalization
- Optimize operations and enhance efficiency
- Identify new business opportunities and market trends
- Make more informed decisions based on data-driven insights
- Reduce costs and increase profitability
From optimizing marketing campaigns to predicting customer churn, big data analytics is transforming how businesses operate and interact with their customers. For example, e-commerce companies can use big data to personalize product recommendations, improve website navigation, and target marketing campaigns more effectively. Healthcare providers can leverage big data to analyze patient data, identify potential health risks, and personalize treatment plans. Financial institutions can use big data to detect fraudulent transactions, assess creditworthiness, and manage risk.
However, harnessing the power of big data analytics comes with its own set of challenges. Organizations need to invest in the necessary infrastructure, talent, and expertise to manage and analyze large datasets. Data privacy and security are also major concerns, as businesses need to ensure that sensitive information is protected. Despite these challenges, the potential benefits of big data analytics are undeniable. As the volume and complexity of data continue to grow, organizations that embrace this technology will be well-positioned to thrive in the digital age.
Understanding the Server Requirements for Big Data
Big data is a term used to describe the massive amount of data that is generated by businesses, organizations, and individuals every day. This data can be structured, semi-structured, or unstructured, and it comes from a variety of sources, including web logs, social media, sensors, and mobile devices. Processing and analyzing this data can provide valuable insights that can help businesses make better decisions, improve their operations, and gain a competitive advantage. However, processing and analyzing big data requires significant computational resources, and this is where server requirements come into play.
There are a number of server requirements that are essential for managing big data. These requirements can be broadly categorized into the following:
Storage
Big data requires a lot of storage space, as the volume of data can be enormous. The type of storage required will depend on the type of data being stored, the frequency of access, and the performance requirements. For example, data that is frequently accessed may be stored on solid-state drives (SSDs) for faster performance, while data that is rarely accessed may be stored on hard disk drives (HDDs).
In addition to the type of storage, the amount of storage space required will also be a major consideration. Many big data applications require petabytes or even exabytes of storage, which can be a significant investment.
Processing Power
Big data requires significant processing power to analyze and process the large amounts of data. The type of processing power required will depend on the type of analysis being performed, the complexity of the algorithms, and the volume of data being processed. Modern CPUs and GPUs are often used for high-performance computing and parallel processing.
Memory
Big data applications often require a large amount of memory to hold the data that is being processed. The amount of memory required will depend on the size of the data set, the complexity of the analysis, and the number of users accessing the data. In many cases, RAM (Random Access Memory) is used for rapid processing and accessing data.
Networking
Big data applications often involve transferring large amounts of data between servers, which can put a strain on the network. High-speed network connections are critical to ensure that data can be transferred quickly and efficiently. Many systems use fiber optic cables for ultra-fast transmission speeds.
Security
Big data contains sensitive information, so it is important to secure the data from unauthorized access. Security measures should include firewalls, intrusion detection systems, and access control mechanisms.
Scalability
Big data applications need to be scalable, which means that they can handle increasing amounts of data as the business grows. This requires using a distributed computing framework, which allows data to be processed across multiple servers.
Availability
Big data applications should be highly available, which means that they are always accessible to users. This can be achieved by using redundant servers and fault-tolerant architectures.
Cost
The cost of managing big data can be significant. It is important to choose the right hardware and software to minimize costs while still meeting the needs of the business. There are options for cloud-based solutions which can be more affordable than on-premises solutions.
By understanding these server requirements, businesses can ensure that they have the right infrastructure in place to manage their big data effectively. Investing in the right hardware and software can help businesses gain valuable insights from their data and achieve their business goals.
Key Considerations When Choosing a Server Solution
In today’s digital landscape, a reliable server solution is paramount for businesses of all sizes. Whether you’re running a small website or a complex enterprise application, choosing the right server can significantly impact your performance, security, and overall success. With so many options available, it’s essential to consider key factors to make an informed decision.
1. Performance and Scalability:
Assess your current and future needs. Consider the traffic volume, processing power, and storage requirements for your applications. If your business is experiencing rapid growth, opt for a scalable server solution that can easily accommodate future demands. Look for servers with robust processors, ample RAM, and sufficient storage capacity.
2. Security:
Data security is crucial. Choose a server with built-in security features such as firewalls, intrusion detection systems, and encryption. Consider using a reputable hosting provider with strong security protocols and data backup options. Implement strong passwords and regularly update your server’s software and security patches.
3. Cost:
Budget is always a factor. Server solutions can vary significantly in price, from affordable cloud servers to expensive on-premises systems. Consider the ongoing costs, such as hardware maintenance, software licenses, and power consumption. Factor in the cost of managing and supporting the server infrastructure.
4. Reliability and Uptime:
Choose a server solution that prioritizes reliability and uptime. Look for providers with a proven track record and strong service level agreements (SLAs). Consider redundant power supplies, backups, and disaster recovery plans to minimize downtime.
5. Support and Management:
Evaluate the level of support and management offered by the server provider. Do they offer 24/7 technical support? Are there managed services available for tasks like server monitoring and updates? Having reliable support can significantly reduce your workload and ensure smooth operation.
6. Flexibility and Customization:
Consider your future needs and the flexibility of the server solution. Can it be easily configured and customized to meet your evolving requirements? Look for options that offer flexibility in terms of operating systems, software installations, and network configurations.
7. Environmental Impact:
For environmentally conscious businesses, consider the server’s power consumption and energy efficiency. Choose a provider that prioritizes sustainable practices and utilizes energy-efficient hardware and cooling solutions.
By carefully considering these factors, you can choose a server solution that meets your specific needs, budget, and long-term goals. Remember, a well-chosen server can be a valuable asset for your business, providing the foundation for growth, innovation, and success.
Top Server Solutions for Big Data Analytics in 2024
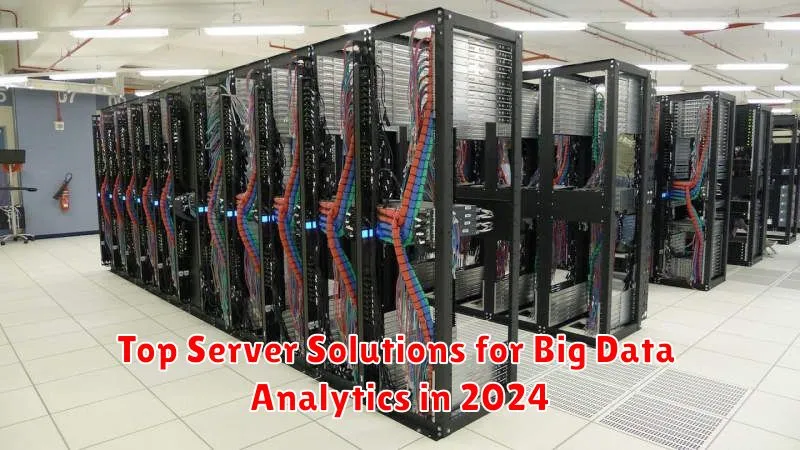
The world is generating data at an unprecedented rate, and organizations are increasingly turning to big data analytics to make sense of it all. To handle the vast amounts of data and perform complex computations, organizations need robust server solutions.
In 2024, there are several server solutions that stand out for their ability to handle big data analytics workloads. Here are some of the top contenders:
1. Cloud-Based Server Solutions
Cloud computing has revolutionized how organizations handle their IT infrastructure. With cloud-based server solutions, businesses can scale their resources up or down as needed, paying only for what they use. This makes them particularly attractive for organizations dealing with large datasets. Some of the leading cloud providers offering server solutions for big data analytics include:
- Amazon Web Services (AWS): AWS offers a wide range of services for big data analytics, including Amazon EMR, Amazon Redshift, and Amazon Athena. These services provide the necessary tools and infrastructure for processing, analyzing, and visualizing data at scale.
- Microsoft Azure: Azure also boasts a comprehensive suite of services for big data analytics, such as Azure Data Lake Analytics, Azure Cosmos DB, and Azure Databricks. These services are designed to handle massive datasets and provide insights into business operations.
- Google Cloud Platform (GCP): GCP offers a variety of services for big data analytics, including Google BigQuery, Google Cloud Dataproc, and Google Cloud Dataflow. These services provide the scalability, performance, and security required for processing large amounts of data.
2. On-Premise Server Solutions
For organizations with strict data security requirements or the need for complete control over their infrastructure, on-premise server solutions may be a better choice. Several vendors offer hardware and software solutions tailored to handle big data analytics workloads. Some of the prominent players in this space include:
- Dell EMC: Dell EMC provides a wide range of server solutions, including the PowerEdge line, which is specifically designed for big data analytics. These servers offer high performance, scalability, and reliability, making them suitable for demanding workloads.
- Hewlett Packard Enterprise (HPE): HPE also offers a range of server solutions for big data analytics, including the ProLiant line. These servers are designed to handle large datasets and provide the necessary computing power for complex analytics tasks.
- IBM: IBM provides a range of hardware and software solutions for big data analytics, including the Power Systems line. These systems are designed to deliver high performance and scalability, enabling organizations to process and analyze large datasets quickly and efficiently.
3. Specialized Big Data Analytics Servers
In addition to general-purpose servers, some vendors offer specialized servers specifically designed for big data analytics. These servers often come equipped with hardware and software optimized for handling the unique demands of these workloads. Some of the key players in this space include:
- Cloudera: Cloudera offers a comprehensive platform for big data analytics, including a suite of software and hardware solutions. Cloudera’s server solutions are designed to deliver high performance and scalability, making them suitable for demanding analytics workloads.
- Hortonworks: Hortonworks offers a range of software and hardware solutions for big data analytics. Their server solutions are optimized for performance and efficiency, enabling organizations to process and analyze large datasets quickly and efficiently.
- MapR Technologies: MapR Technologies offers a distributed file system and a range of software and hardware solutions for big data analytics. Their server solutions are designed for high performance and scalability, making them suitable for demanding analytics workloads.
Choosing the Right Server Solution
The best server solution for big data analytics will depend on several factors, including:
- Data Volume and Complexity: The size and complexity of the data will determine the processing power and storage capacity required.
- Performance Requirements: The speed at which insights need to be generated will influence the choice of server solution.
- Budget: The cost of hardware and software will be a key consideration for organizations of all sizes.
- Security and Compliance: Organizations with strict security requirements may need to consider on-premise server solutions.
By carefully evaluating their specific needs and requirements, organizations can choose the right server solution to support their big data analytics initiatives and unlock valuable insights from their data.
Cloud-Based Servers for Scalability and Flexibility
In today’s fast-paced digital world, businesses need to be agile and adaptable to succeed. Traditional on-premises servers often struggle to keep up with the demands of modern applications and fluctuating workloads. This is where cloud-based servers come into play, offering a solution that prioritizes scalability and flexibility.
Cloud servers, also known as virtual servers, run on a network of data centers and are accessed over the internet. This allows businesses to scale their computing resources up or down as needed, without the limitations of physical hardware. With cloud servers, you can easily adjust your server capacity based on traffic spikes, seasonal demands, or unexpected growth.
Benefits of Cloud-Based Servers
Here are some key benefits of using cloud-based servers:
- Scalability: Easily adjust your server resources to meet changing demands.
- Flexibility: Access a wide range of server configurations and operating systems.
- Cost-Effectiveness: Pay only for the resources you use, eliminating upfront investments in hardware.
- High Availability: Redundant servers ensure minimal downtime and data loss.
- Security: Benefit from robust security measures and regular updates.
- Global Reach: Access servers from anywhere in the world.
Types of Cloud Servers
There are different types of cloud servers available, each offering unique benefits:
- Virtual Private Servers (VPS): Dedicated resources within a shared environment.
- Dedicated Servers: Entire servers dedicated to your needs, offering maximum performance and control.
- Containerized Servers: Lightweight and portable servers that simplify deployment and management.
Conclusion
Cloud-based servers provide a powerful solution for businesses seeking scalability, flexibility, and cost-effectiveness. By leveraging the cloud, you can optimize your IT infrastructure, enhance performance, and gain a competitive edge in today’s dynamic marketplace.
High-Performance Computing (HPC) Servers
High-performance computing (HPC) servers are specialized computer systems designed for handling complex and computationally intensive tasks. They are typically used in scientific research, engineering, finance, and other industries where speed and efficiency are paramount.
HPC servers are characterized by several key features, including:
- High processing power: Equipped with multiple CPUs, GPUs, or specialized processors, HPC servers deliver exceptional processing speeds.
- Large memory capacity: They often have terabytes or even petabytes of RAM to handle massive datasets and complex simulations.
- High-speed interconnects: Fast networking technologies like InfiniBand or Ethernet ensure seamless data transfer between nodes in a cluster.
- Parallel processing: HPC servers can distribute tasks across multiple processors and nodes, enabling parallel computing for significant speed gains.
- Scalability: HPC clusters can be easily expanded by adding more nodes to meet growing computational demands.
Applications of HPC Servers
HPC servers find applications in a wide range of fields, including:
- Scientific research: Accelerating simulations, modeling complex systems, and analyzing large datasets in fields like physics, chemistry, biology, and astronomy.
- Engineering: Designing and simulating products, structures, and processes, such as aircraft, automobiles, and buildings.
- Finance: Analyzing market data, running risk simulations, and developing trading algorithms.
- Medicine: Conducting drug discovery research, analyzing medical images, and developing personalized treatments.
- Climate modeling: Predicting weather patterns, understanding climate change, and forecasting natural disasters.
Advantages of HPC Servers
The use of HPC servers offers numerous advantages, including:
- Faster computation times: HPC servers significantly reduce the time required to complete complex tasks.
- Improved accuracy and precision: Parallel processing and large memory capacity enable more detailed and accurate simulations and analyses.
- Enhanced productivity: By automating tasks and speeding up processes, HPC servers increase overall productivity.
- New insights and discoveries: The ability to process vast amounts of data and run complex simulations opens up new avenues for research and innovation.
- Competitive advantage: HPC servers can give businesses a competitive edge by enabling them to develop new products and services faster and more efficiently.
Choosing the Right HPC Server
Selecting the appropriate HPC server depends on the specific computational needs of an organization or project. Factors to consider include:
- Computational workload: The type and complexity of tasks that need to be performed.
- Data size and volume: The amount of data to be processed and stored.
- Budget and resources: The financial constraints and available infrastructure.
- Scalability requirements: The need to expand the system in the future.
With their exceptional processing power, large memory capacity, and parallel processing capabilities, HPC servers are essential tools for tackling computationally intensive tasks in various fields. They empower researchers, engineers, and businesses to unlock new insights, push the boundaries of innovation, and achieve breakthroughs that were previously impossible.
GPU Servers for Accelerated Data Processing
In the era of big data, processing vast amounts of information quickly and efficiently is paramount. Traditional CPU-based servers often struggle to keep pace with the demands of modern applications, leading to bottlenecks and delays. This is where GPU servers come into play, offering a powerful solution for accelerated data processing.
GPUs (Graphics Processing Units), originally designed for rendering graphics, have evolved into highly parallel processing engines. Unlike CPUs, which excel at sequential tasks, GPUs are optimized for handling massive numbers of calculations simultaneously. This parallel processing capability makes them ideal for data-intensive tasks, such as machine learning, deep learning, scientific simulations, and high-performance computing.
GPU servers are designed with multiple GPUs integrated into their hardware. This allows for substantial increases in processing power, enabling applications to execute significantly faster. Here are some key benefits of using GPU servers for accelerated data processing:
- Increased Processing Speed: GPUs handle parallel computations efficiently, leading to dramatic performance gains for data-intensive tasks.
- Enhanced Machine Learning: GPU servers are essential for training and deploying complex machine learning models, accelerating the development and deployment of AI applications.
- Scientific Computing: Simulations and complex scientific calculations can be performed much faster with the help of GPUs, enabling researchers to make breakthroughs.
- Big Data Analytics: GPUs allow for faster analysis of large datasets, providing insights and patterns that would be difficult to uncover otherwise.
While GPU servers offer significant advantages, it’s important to consider their limitations. The cost of GPU servers can be higher than traditional servers. Additionally, specific software and expertise are required to effectively utilize GPU acceleration.
In conclusion, GPU servers are a game-changer for accelerated data processing. Their powerful parallel processing capabilities enhance performance, accelerate data analysis, and enable the development of cutting-edge applications. As data volumes continue to grow exponentially, GPU servers will play an increasingly crucial role in unlocking the potential of big data and driving innovation across various industries.
Data Center Infrastructure Management (DCIM) Software
Data center infrastructure management (DCIM) software is a suite of tools that helps organizations manage their data center infrastructure, including servers, storage, networking devices, power, cooling, and security. DCIM software can help organizations improve the efficiency and effectiveness of their data centers by providing a centralized view of all of their infrastructure components.
DCIM software can be used to monitor and manage a wide range of data center infrastructure components, including:
- Servers
- Storage devices
- Networking devices
- Power distribution units (PDUs)
- Cooling systems
- Security systems
DCIM software can also be used to automate a number of tasks, such as:
- Provisioning new servers
- Monitoring server performance
- Managing power consumption
- Controlling environmental conditions
- Generating reports
The benefits of using DCIM software include:
- Improved efficiency: DCIM software can help organizations improve the efficiency of their data centers by automating tasks and providing a centralized view of all of their infrastructure components.
- Reduced costs: DCIM software can help organizations reduce costs by optimizing power consumption, reducing downtime, and improving utilization rates.
- Increased availability: DCIM software can help organizations improve the availability of their data centers by providing real-time monitoring and alerting capabilities.
- Enhanced security: DCIM software can help organizations enhance the security of their data centers by providing access control and monitoring capabilities.
- Improved compliance: DCIM software can help organizations comply with industry regulations by providing audit trails and reporting capabilities.
DCIM software is an essential tool for any organization that wants to manage its data center infrastructure effectively. By providing a centralized view of all of their infrastructure components, DCIM software can help organizations improve the efficiency, effectiveness, and security of their data centers.
Optimizing Server Performance for Big Data Workloads
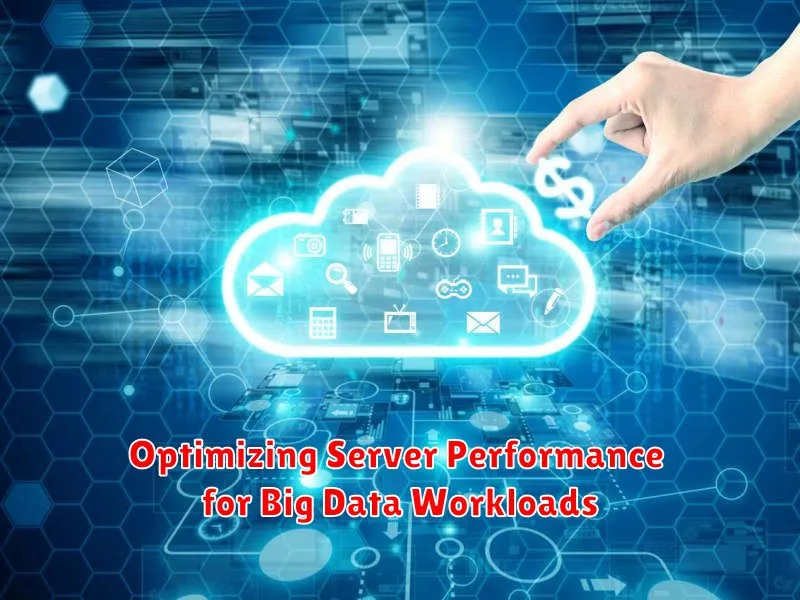
Handling big data workloads efficiently is crucial for organizations across various industries. With the exponential growth of data, optimizing server performance is essential to ensure smooth processing, analysis, and decision-making. This article explores key strategies for optimizing server performance to efficiently handle big data workloads.
1. Hardware Optimization
The foundation of high-performance servers lies in robust hardware components. Here’s a breakdown of crucial aspects:
- Processors (CPUs): Opt for multi-core processors with high clock speeds to handle complex computations efficiently. Consider CPUs with specialized instructions like AVX for accelerated data processing.
- Memory (RAM): Big data workloads demand ample RAM to hold large datasets in memory, reducing disk access and improving performance. Choose servers with sufficient RAM based on the data size and processing requirements.
- Storage: Use high-performance storage solutions like NVMe SSDs for rapid data access. Consider using distributed storage systems for large datasets, ensuring scalability and fault tolerance.
- Network Interface Cards (NICs): Ensure high-speed network connectivity to enable fast data transfer between servers and other components.
2. Software Optimization
Software plays a vital role in optimizing server performance. Here are key areas to focus on:
- Operating System (OS): Choose an OS specifically designed for high-performance computing, such as Linux distributions like CentOS or Ubuntu Server. Configure the OS for optimal resource utilization and minimal overhead.
- Database Management System (DBMS): Select a DBMS that’s optimized for handling large datasets, such as PostgreSQL, MySQL, or MongoDB. Consider using columnar databases for faster analytical queries.
- Big Data Frameworks: Leverage big data frameworks like Hadoop, Spark, or Hive for distributed processing and analysis of large datasets. Optimize these frameworks for efficient resource utilization and data flow.
- Caching: Implement caching mechanisms to store frequently accessed data in memory, reducing disk access times and improving response times.
3. Infrastructure Optimization
Proper infrastructure design and management are crucial for optimal performance. Consider these factors:
- Server Clusterization: Deploy servers in clusters to distribute workloads, enhance scalability, and improve fault tolerance. Utilize load balancing mechanisms to distribute traffic evenly across servers.
- Network Optimization: Ensure high-speed network connections and efficient network configuration to minimize latency and data transfer bottlenecks.
- Monitoring and Logging: Implement robust monitoring tools to track server performance metrics, identify bottlenecks, and proactively address performance issues.
- Security Measures: Implement appropriate security measures to protect the servers and data from unauthorized access and attacks. Regular security updates and patches are essential.
4. Data Optimization
Optimizing the data itself can significantly impact server performance. Here are some techniques:
- Data Compression: Compress data before storing it to reduce storage space and improve data transfer speeds. Use appropriate compression algorithms based on the data format.
- Data Deduplication: Identify and eliminate duplicate data entries to reduce storage space and improve query performance. Implement data deduplication techniques at the storage or application level.
- Data Partitioning: Split large datasets into smaller partitions to improve processing efficiency. Distribute partitions across multiple servers for parallel processing.
Conclusion
Optimizing server performance for big data workloads requires a comprehensive approach that considers hardware, software, infrastructure, and data optimization strategies. By implementing these best practices, organizations can ensure efficient processing, analysis, and decision-making, harnessing the power of big data for meaningful insights and competitive advantage.
Future Trends in Big Data Server Solutions
The realm of big data is constantly evolving, with new technologies and trends emerging at a rapid pace. This dynamism demands a continuous adaptation of server solutions to handle the increasing volume, velocity, and variety of data. In this context, several key trends are shaping the future of big data server solutions, paving the way for enhanced performance, scalability, and efficiency.
Cloud-Native Architecture
Cloud-native architectures are becoming increasingly prevalent in the big data landscape. These architectures leverage the agility and scalability of cloud platforms, enabling organizations to deploy and manage big data workloads more efficiently. Server solutions are adapting to this trend by offering cloud-optimized features, such as containerization, microservices, and serverless computing, to enhance flexibility and cost-effectiveness.
Edge Computing
Edge computing is gaining traction as a means to process data closer to its source, reducing latency and improving real-time insights. This trend necessitates specialized server solutions at the edge, equipped with powerful processing capabilities and low-latency connectivity. These edge servers can handle initial data processing and analysis, offloading the core data center and enabling faster decision-making.
Artificial Intelligence (AI) and Machine Learning (ML)
The integration of AI and ML into big data server solutions is revolutionizing data analysis and insights extraction. Servers are becoming more intelligent, equipped with specialized hardware and software that accelerate AI and ML workloads. This allows for more sophisticated analytics, predictive modeling, and automated decision-making, driving significant value from big data.
Data Security and Privacy
As the volume and sensitivity of data grow, data security and privacy become paramount. Big data server solutions are evolving to incorporate robust security features, such as encryption, access control, and compliance with regulations like GDPR. This ensures the integrity and confidentiality of data, building trust and confidence in data-driven operations.
Sustainable Server Solutions
The environmental impact of data centers is gaining attention, leading to a focus on sustainable server solutions. Energy-efficient hardware, optimized cooling systems, and renewable energy sources are becoming integral aspects of big data infrastructure. This trend promotes responsible data processing while reducing operational costs.
Conclusion
The future of big data server solutions is characterized by innovation, efficiency, and sustainability. As technology continues to advance, these trends will further shape the landscape, enabling organizations to harness the power of big data effectively, drive better decision-making, and unlock new opportunities for growth and innovation.